A couple of weeks ago I asked you to rate an interview question that we had sent to candidates. The idea is to build a persistent phone book, with the idea that we care about the amount of I/O traffic that is used more than anything else.
The scenario we presented to the candidates was:
The rules are that you can’t maintain any state in the class itself and that the code should be prepared to handled a lot of records. Of particular interest is the IterateOrderedByName() call, which allows you to do an ordered iteration (by name) from a given name. That pretty much forces us to store the data in a sorted format.
Note that we don’t explicitly state that in the requirements for the task, we expect the candidates to understand that this is a requirement given the requirements for the operations. The most naïve option to complete this challenge is to write a CSV file. Each entry in its own line and you are done. However, that wouldn’t allow us to meet the other requirements.
In other words, reading the whole file to memory, adding the item, sorting the whole thing and writing it back again is a no go. As a note, this is a task that we give to Junior developers. We expect zero to very little experience.
After going over dozens such answers, I can tell you that this task does its primary job, which is to filter people out. That is an explicit goal, by the way. We have had over 200 applicants to this position and the time it takes to go through the that many CVs, interviews, etc is horrendous. I found that this question filters enough people to make it far more manageable. And given the answers I got to my previous post, this is absolutely the kind of task that I would consider a junior developer suitable for. I remember solving similar problems in high school.
I like this problem because it is a good way to see if a person is able to do several things at once:
- Can take a non trivial problem and break it to manageable pieces.
- Can figure out several pitfalls along the way and handle them.
- Can recognize what the impact of the various requirements are for the solution.
- Can apply knowledge from one field to another. In this case, in memory data structures vs. persistent files.
- Understand that data and representations are different things.
I have to admit that I was quite surprised by the results. Pretty much no one chose to use binary format, they all went to the textual format. This makes the problem harder. Also, there were a number of bytes vs. chars errors (that isn’t an issue for a junior position, though).
My first attempt is going to be a bit brute force. Instead of trying to implement any fancy data structures, I’m going to simply write the data out to the file in an append only manner. At the end of the file, however, I’ll keep a sorted array of the positions of the items in the file. Here is what this looks like:
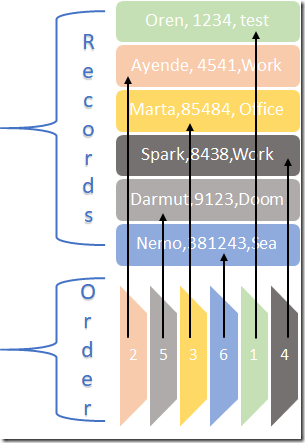
To do a search in this format, I can use the sorted positions at the end of the file. Whenever I add a new record, I add it at the end of the records (overwriting the sorted positions sections and then writing the new sorted positions at the end). The overhead per write is the size of the sorted array, basically. The bigger the file, the more we’ll spend writing to the array. For example, assuming each record is around 64 bytes, when we get to 10 million records, we’ll have data size of 610MB, but the metadata we’ll have will be around 38 MB (and we’ll need to write all of it each time we modify a record).
The advantages, however, is that the code is simple and there are several ways that we can optimize the code without too much trouble. Here is what this looks like in code, there are some tests attached that you can run and the entire code runs at roughly 150 lines of code.
That isn’t a great solution, I’ll admit, but it is a straightforward one. It seems like it would be the natural consequence of moving from appending each record to the file to having a sorted access. There are some nice things there, nevertheless. You can “batch” several inserts together and close them by calling Flush() once, instead on each record, for example.
Given that the biggest issue here is the size of the sorted positions, we can seek to reduce it in several ways:
- We can compress the data on write, you can see the changes required for that here.
- We can create multiple sorted positions and merge them occasionally.
For that matter, we can store the sorted positions in another file entirely, which will simplify things like supporting efficient in order inserts.
Things that a junior developer might not do here? I’m using BinaryReader and BinaryWriter. That simplify things for me, since I can ignore the cost of textual parsing. That said, I’m not using them in any interesting way and the concepts translates 1:1 to textual interface, with a bit more work.
Can you do better than this implementation?